The pharmaceutical industry stands at a pivotal crossroads.
Known for groundbreaking advancements in medicine and health, the sector has been slow in digital adoption. This has negatively impacted business operations and value delivery.
Dismissing digital transformation presents big risks to pharmas. Notably, it challenges the industry’s ability to remain competitive and responsive to evolving healthcare demands.
Historically, the pharmaceutical sector’s focus has been mainly on drug discovery and development.
Though pharma companies deal with extensive research cycles, strict regulatory demands, and hefty R&D investments, these steps are vital to launch new, lifesaving drugs.
However, this focus often overshadows innovation in other business areas. It also leads to a reliance on legacy systems and traditional methodologies resistant to change.
The slow embrace of digital transformation in pharma is fraught with risks:
- Pharmaceutical companies risk being outpaced by more digitally agile competitors who innovate rapidly and bring new drugs to market faster.
- Failing to meet consumers’ evolving expectations, especially in an era where digital health solutions are increasingly preferred, is a big concern.
- A lack of digital agility can hinder the industry's response to global health crises (see: COVID-19), which demand swift adaptation and innovation.
Fortunately, artificial intelligence offers a powerful catalyst for the pharmaceutical industry. It enables innovation beyond drug discovery and into broader operations and the value chain.
The use of AI empowers tech entrepreneurs, in particular, to address the industry's big challenges. With AI, business leaders can make healthcare more accessible, effective, and attuned to diverse patient needs.

Artificial intelligence in the pharmaceutical industry: Evaluating emerging opportunities
To assess AI’s potential, we’ve developed a framework inspired by the team at Emergence Capital and validated by industry and technical experts focusing on three dimensions:
1) AI enablement (10x improvement)
This dimension assesses how AI could improve productivity and customer experience, aid with competitive differentiation, and lead to a quantifiable ROI for today’s pharmas.
2) Complexity of human task (poised to be automated)
Here, we evaluate the complexity of tasks within the jobs. Tasks performed by experts are deemed high-complexity, while routine tasks are low-complexity (but by no means insignificant).
3) Data readiness
In pharma, data often exists in silos and across structured and unstructured systems. For example, electronic medical records may reside in multiple, disconnected solutions.
A big hurdle for AI adoption is usable-data availability and accessibility.
We recognize the challenges of data cleaning and integration. Yet advancements in AI and large language models make the processing of unstructured data increasingly feasible.
Consider the cost, integration, and time factors impacting your data readiness.

Our AI-in-pharma framework: How to deploy artificial intelligence in 6 distinct ways
Knowing the value of AI in pharma outside early-stage drug discovery and clinical development is one thing. Learning how your pharmaceutical company can explore and take advantage of these other opportunities for innovation to tackle long-standing problems is another.
We collaborated with subject matter experts at Eli Lilly and BioGen and various venture capital firms to identify six critical functions within the pharmaceutical value chain ripe for AI disruption.
Specifically, functions that can lead to the next wave of pioneering, venture-backed startups.
Let's examine these opportunity areas.
1) Clinical trials
Clinical trials help get new medicine approved for use. But the current clinical trial landscape is marked by inefficiencies. Problems tied to drug candidate enrollment, protocol optimization, data management, and diversity and inclusion remain prevalent.
Together, these issues contribute to prolonged timelines and escalated costs.
PAREXEL VP, Patient Engagement Rosamund Round recently told BioPharma Dive 85% of all clinical trials fail to recruit enough patients. The resulting financial impact of these delays can be massive.
AI in pharma use cases
- Accurate data collection, storage, and analysis. The pharmaceutical industry needs help managing large data volumes. Mixing manual and digital data organization processes lead to consistencies. With AI, pharma companies can automate data handling to increase accuracy and speed of access to real-time clinical trial analytics. This results in fewer data collection errors and more efficient storage and advanced analysis. It also enhances drug development reliability and post-launch drug efficacy and safety monitoring and ensures regulatory compliance.
- Diversity in clinical trials. Traditional methods often fail to ensure diverse clinical trial participation, which is vital for representative drug efficacy and safety data. The use of AI-driven analytics can identify and target underrepresented groups, leading to more inclusive trials. This approach improves the diversity and reliability of clinical trial data and overall drug development outcomes by tailoring communication and outreach to diverse populations.
2) Regulatory affairs
The role of pharmacovigilance and post-market side effect monitoring cannot be overstated.
More than 1 million reports are logged annually in the FDA Adverse Event Recording System. And research featured in Frontiers in Pharmacology shows 3.2% of hospitalized patients experienced adverse drug reactions (ADRs) during their stay, leading to many deaths annually and costing healthcare providers and pharma companies billions of dollars.
AI in pharma use cases
- Post-marketing surveillance compliance. Tracking the ongoing safety of drugs and monitoring efficacy in the real world is difficult. That said, AI can aid in ensuring drug safety and efficacy post-launch. It has the potential to streamline the detection of ADRs, which can enhance reporting efficiency and ensure regulatory compliance.
- Pharmacovigilance and patient safety. Pharmacovigilance involves complex data collection to detect, assess, and prevent adverse side effects. With its advanced data analytics and machine learning capabilities, AI could process clinical trials and patient record data swiftly and accurately. This is a crucial step in building patient trust in the pharmaceutical industry.
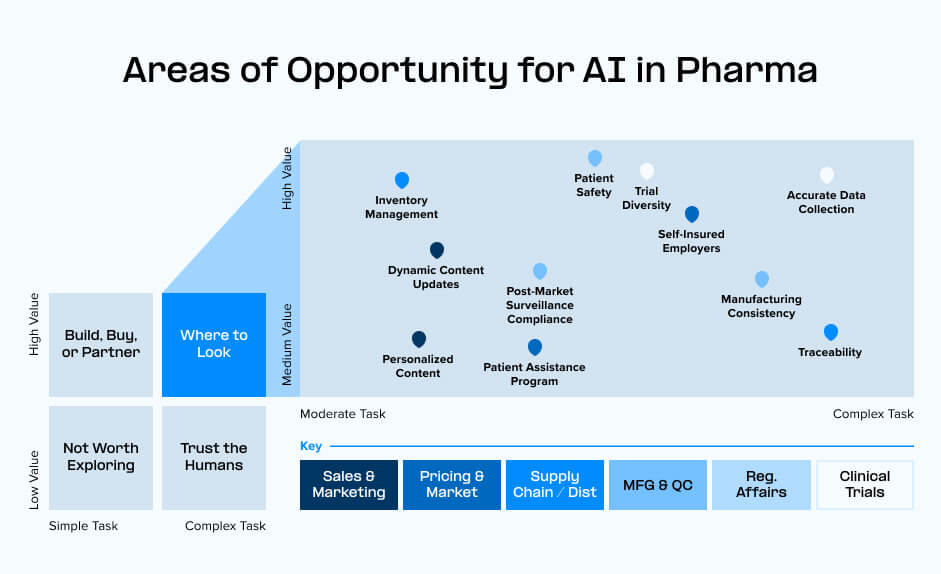
3) Manufacturing and quality control
Quality lapses in drug manufacturing can have severe consequences, including ADRs, eroded patient trust, treatment disruptions, and mental health impacts.
In 2022, the FDA reported 166 manufacturing sites responsible for 912 drug recalls. These recalls can cost up to $600 million each and are commonly due to missed rare side effects, healthier-than-average study subjects, manufacturing issues, and manufacturer misconduct.
AI in pharma use cases
- Ensuring consistent quality. While rigorous, traditional quality control methods within the manufacturing process are often time-consuming and prone to human error. The integration of AI offers an opportunity to enhance the precision of efficiency in quality assurance. With AI's ability to continuously monitor drug production and analyze data, there is the potential to ID and correct inconsistencies quickly. This means a lower likelihood of recalls and better patient safety.
4) Supply chain and distribution
The pharmaceutical industry, greatly impacted by COVID-19, has encountered challenges adapting to rapidly changing drug demand and supply dynamics.
This situation, worsened by global supply chain disruptions and diabetic medication shortages due to off-label weight loss usage, underscores the urgent need for more effective inventory management and traceability systems.
A recent nVentic white paper reveals that, in 2022, pharma supply and demand inefficiencies led to approximately $12.5 billion in losses for 28 analyzed companies.
This highlights the need for improved responsiveness in the sector.
AI in pharma use cases
- Inventory management and optimization. Managing inventory efficiently is a big challenge. Traditional systems often fail to forecast demand. This leads to stockouts or overstocking. But AI tech can help by utilizing algorithms to analyze data trends and optimize drug stock levels. This can reduce shortages, waste, and costs, improve service levels, and free up working capital.
- Traceability. Traceability is vital for drug safety and authenticity. It ensures meds can be tracked from production to patients. With advanced AI tracking systems, potentially integrated with blockchain tech, the drug supply chain can be monitored in real time. That means improved tracking accuracy and efficiency in managing recalls and combating issues like counterfeit drugs.
5) Pricing and market access
Prescription medication affordability is a complex issue in today's healthcare system.
A Kaiser Family Foundation report reveals nearly 30% of U.S. adults skipped prescribed meds in 2019 due to cost. This problem was exacerbated for those lacking comprehensive insurance.
Meanwhile, self-insured employers, covering about 153 million Americans, face challenges.
Despite customizing health benefits using employee data, a Health Affairs study shows healthcare spending for those in self-insured plans is about 10% higher than in fully insured plans.
This indicates a need for strategy evolution among these employers.
AI in pharma use cases
- Patient assistant programs (PAPs). These programs help lower-income households access inexpensive meds. But PAPs must be run efficiently and made accessible to this broad consumer segment. Integrating AI into PAPs can streamline processes, pinpoint eligible patients more effectively, and tailor assistance to individual needs. The spillover impact is substantial. By improving patient adherence, pharmas can chip away at challenges tied to inventory management and optimization.
- Self-insured employers. Artificial intelligence enables these employers to pursue the next logical step in their evolution: assuming a more direct role in health insurance and pharmaceutical management. By doing so, they can negotiate directly with care providers and drug manufacturers and bypass traditional intermediaries. This direct dialogue can lead to more cost-effective healthcare solutions. That's because these employers can gain control over healthcare spending and ensure more favorable terms based on their specific employee population's needs.

6) Sales and marketing
The evolving pharma landscape demands efficient dissemination of info and personalized content to meet diverse consumer needs. That's where marketing and sales come into play.
Traditional sales and marketing approaches are becoming less effective, as medical knowledge and patient requirements rapidly change.
The U.S. pharmaceutical industry has faced billions of dollars in penalties for non-compliance with FDA standards in the past few decades.
The industry must now navigate the dual challenge of updating healthcare professionals and patients with the latest info while also customizing it to individual preferences.
Providing dynamic updates and sharing personalized content using AI-enabled tech can empower pharmas to better connect with these two audiences across the continuum of care.
AI in pharma use cases
- Dynamic content updates. Pharmaceutical marketing teams can use AI to automate content updates across digital platforms in real time. This enhances the reliability and relevance of info, ensuring that healthcare professionals and patients always have access to the latest data.
- Personalized content. What's more, AI can tailor healthcare content to individual patient profiles, factoring in their data, preferences, and history. This personalization leads to improved patient engagement and understanding, better adherence to treatment plans, overall healthier outcomes, and a more inclusive and responsive healthcare system that caters to each patient's unique needs.
Exploring AI tech venture creation — and embracing innovation — at your pharma
The pharmaceutical industry is an intricate, constantly changing landscape. But it's increasingly clear AI presents a rapid acceleration that requires a balance of exploration and risk mitigation.
From enhancing clinical trials and regulatory compliance to transforming manufacturing, supply chain management, and patient-centric marketing, AI is a beacon of growth, efficiency, and progress.
The opportunities are vast and varied. Artificial intelligence can tackle current challenges and create a more accessible healthcare industry that meets the needs of a diverse patient population.
We invite pharmaceutical companies to join High Alpha Innovation in this exciting journey.
Together, we can explore the uncharted territories of AI-enabled innovation, creating solutions that are transformative, sustainable, and compliant with the highest regulatory standards.